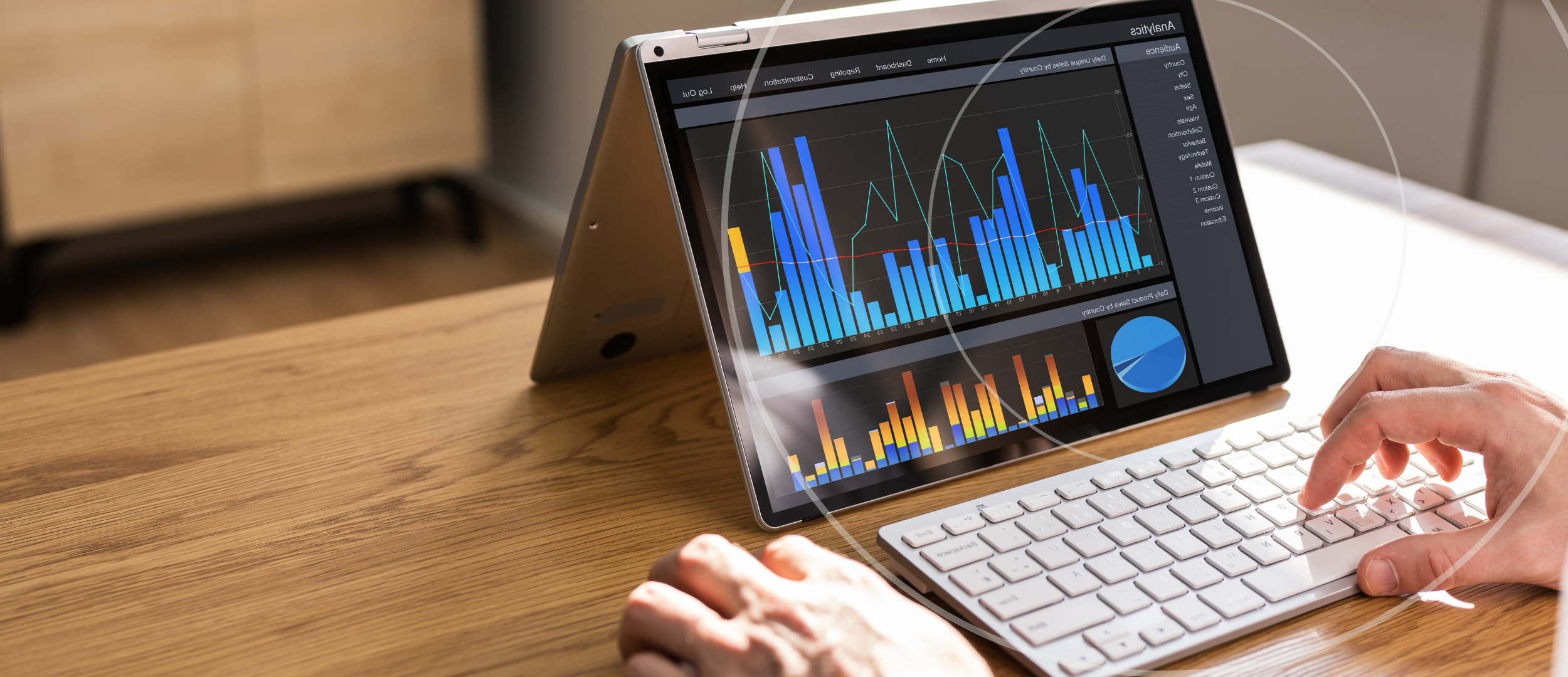
CASE STUDY
derry city & strabane district council
Derry City & Strabane District Council (DCSDC) engaged izon with two eyes planted firmly on the future. As a council, they have committed to reducing their carbon footprint, and wanted to prove a model for renewable, sustainable energy within the council, in anticipation of the core grid architecture modernising in the area.
Our models were developed to accurately predict:
cost
demand
generation
carbon/renewable fuel mix
Complex and complete modelling of the current council architecture and generation sources had to be considered, along with the variables inherent within some renewable energy generation streams. The aim of the project was to enable the council not only to ascertain the current generation capabilities, but also to advise on infrastructure investment requirements to hit their sustainable energy goals, whilst keeping an eye on evolving market trends, such as EV charging points.
To achieve this we developed machine learning and AI algorithms to predict next day cost and weather features to provide the council with the most accurate possible predictions. There were numerous challenges presented using the general data available, owing to the lag between recording and public availability, and advanced algorithms had to be developed to mediate the inaccuracies of historical data. Over a period of 3 months, we applied and tested various models that allowed us to predict to the closest possible degree of accuracy for review for the council.
Many challenges arose over the availability of datasets available, compounded by Covid-19 as on-demand data for individual buildings was patchy due to lack of use, and historical data was not necessarily representative of 2022 data. To overcome this we built models factoring in these variables, and we worked with the hyperparameters to ascertain the most accurate view of usage. Going forward we are working to retrain the machine learning to make sure that the datasets continue to be as accurate as possible,
The model built by izon factors in live condition data from OikoLab (weather metrics), Single Electricity Market Operator/SEMO (Electricity price and volume metrics), and Eirgrid (fuel mix modelling), to give the council the most accurate model of their needs. Solar generation was predicted utilising a Random Forest model using data collected from within the council.
Factoring in a biogas plant, turbines, and solar, has allowed DCSDC to fully evaluate their fuel mix across all 22 buildings to understand the best way to reduce their carbon footprint and evaluate their needs and put a strategy in place for a reduction in non-renewable energy sources for the council.
The model continues to run and is providing invaluable strategic information to the council as they continue to invest in a leading renewable energy strategy, informed by an accurate, end to end view of capabilities and demand.
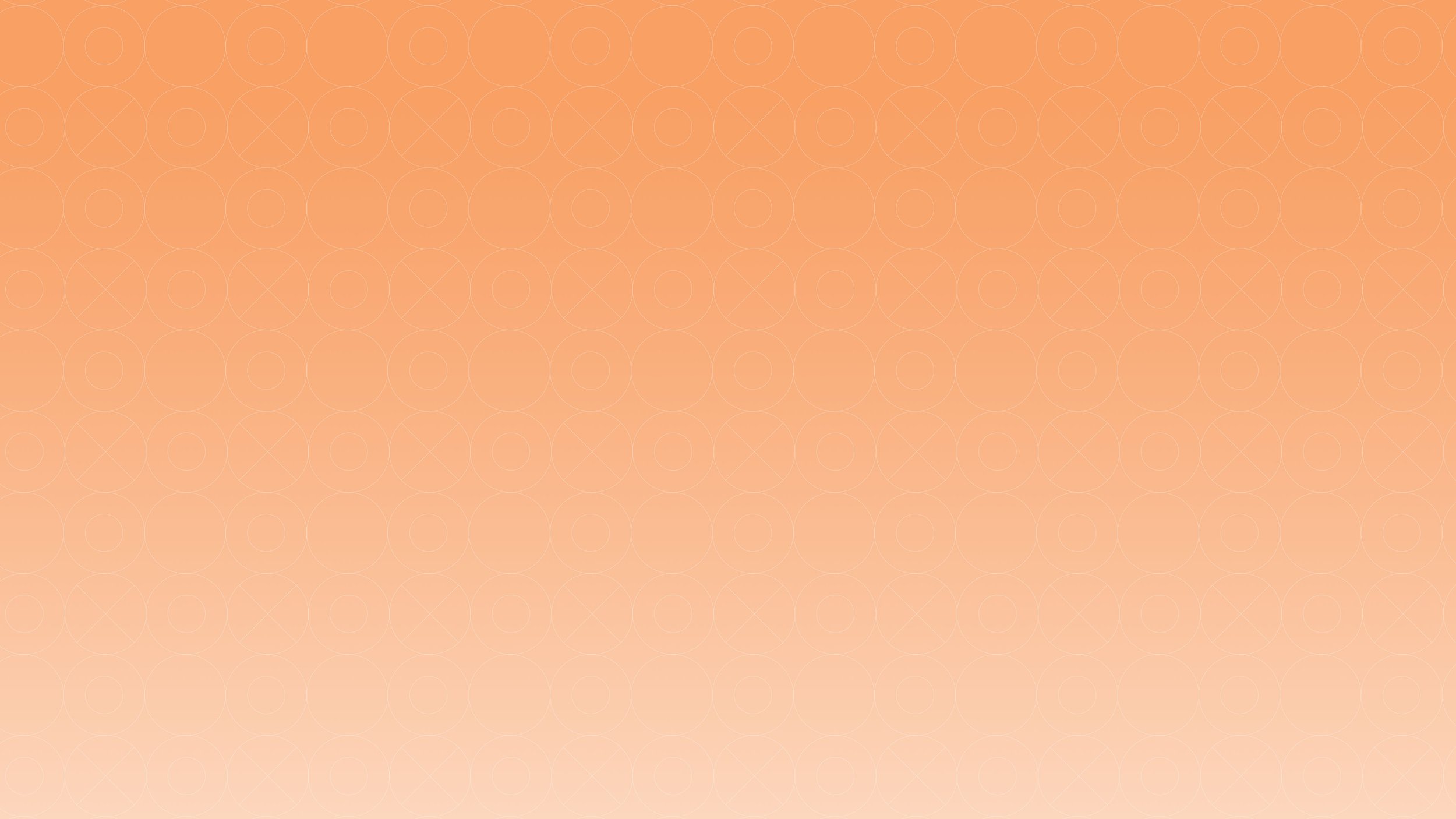
INTERESTED IN REQUESTING A DEMO?
Get in contact with our sales team today.